Objectives
We seek
to evaluate the accuracy of computational intelligence (CI)
methods in time series forecasting, extending the earlier
NN3 forecasting competition unto a new set of daily data.
We hope to
evaluate progress in modelling neural networks for forecasting & to disseminate
knowledge on “best practices”. The competition is conducted for academic purposes
and should not be exploited commercially.
Methods
The prediction competition is open to all methods of computational
intelligence, incl. feed-forward and recurrent neural networks,
fuzzy predictors, evolutionary & genetic algorithms, decision & regression tress, support vector
regression, hybrid approaches etc. used in financial forecasting,
statistical prediction, time series analysis. We also welcome
submission of statistical methods as benchmarks, but they
are not eligible to "win" the NN5.
Dissemination &
Publication
of Results
All those submitting predictions will be invited to
participate in sessions at the 2008 International
Symposium on Forecasting, ISF'07,
Nice, France, the 2008 IEEE
World Congress on Computational Intelligence, Hong Kong,
China, held simultaneously with the
2008 IEEE International Joint
Conference on Neural Networks, IJCNN'08, the
2008 Congress on Evolutionary
Computation, CEC'08 and the
2008 International Conference on Fuzzy Systems FUZZ-IEEE'08,
or the
2008 International Conference on Data
Mining, DMIN'08, Las Vegas. Each workshop will provide awards by dataset for students and non-students.
We are currently negotiating with various publishing houses
for a journal special issue for all accepted submissions.
|
Forecasting
Problem
Forecast a set of 11 or 111 daily time series
of cash money
withdrawals
at cash-machines as accurately as
possible, using methods from computational intelligence and
applying a consistent methodology.
The data consists
of 2 years of daily cash money demand at various automatic
teller machines (ATMs, or cash machines) at different
locations in England (see time series & zoom below):
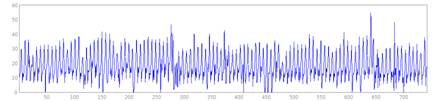
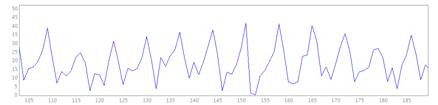
Cash machines
operate as miniature “retail outlets” and provide cash money
to customers. The data may contain
a number of time series patterns including multiple
overlying seasonality, local trends, structural breaks,
outliers, zero and missing values etc. These are often
driven by a combination of unknown and unobserved causal
forces driven by the underlying yearly calendar, such as
reoccurring seasonal periods, bank holidays, or special
events of different length and magnitude of impact, with
different lead and lag effects.
|